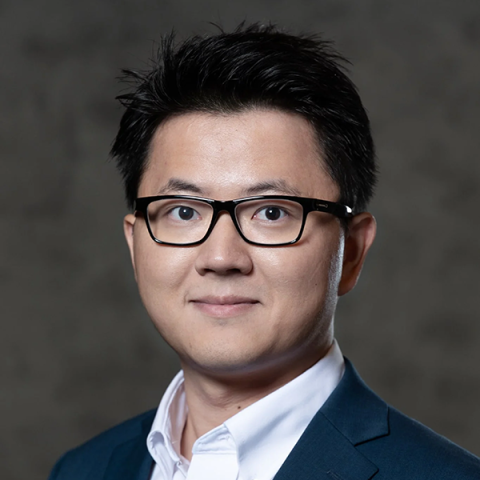
Assistant Professor, Mechanical, Aerospace and Nuclear Engineering
We develop novel efficient, robust and generalizable surrogate modeling techniques for numerical simulations of transport phenomenon. Such models are the key enablers for reducing design cycle and experimental costs.
Bhatnagar, S., Afshar, Y., Pan, S., Duraisamy, K., & Kaushik, S. (2019). Prediction of aerodynamic flow fields using convolutional neural networks. Computational Mechanics, 64, 525–545. Springer Berlin Heidelberg.
Sun, L., Gao, H., Pan, S., & Wang, J.-X. (2020). Surrogate modeling for fluid flows based on physics-constrained deep learning without simulation data. Computer Methods in Applied Mechanics and Engineering, 361, 112732. North-Holland.
Pan, S., & Duraisamy, K. (2020). Physics-informed probabilistic learning of linear embeddings of nonlinear dynamics with guaranteed stability. SIAM Journal on Applied Dynamical Systems, 19(1), 480–509. Society for Industrial and Applied Mathematics.
Ji, W., Qiu, W., Shi, Z., Pan, S., & Deng, S. (2021). Stiff-pinn: Physics-informed neural network for stiff chemical kinetics. The Journal of Physical Chemistry A, 125(36), 8098–8106. American Chemical Society.
Jang, B., Kaptanoglu, A. A., Gaur, R., Pan, S., Landreman, M., & Dorland, W. (2023). Grad-Shafranov equilibria via data-free physics informed neural networks. arXiv preprint arXiv:2311.13491. (accepted in Physics of Plasma)